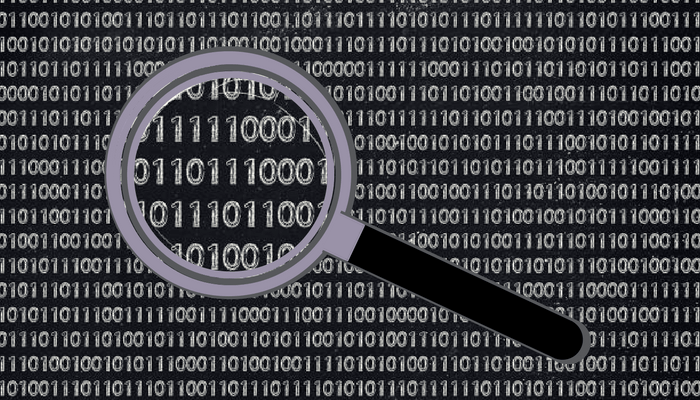
A: There are two approaches related to supply chain planning; the second is “Unsupervised Learning.” (the first was explained here)
The Unsupervised Learning approaches are Similarity Matching and Clustering. These approaches are where the system looks for patterns in the data that appear to have significance, which may point to issues the users would not have identified. In both cases, these approaches “let the data speak” rather than testing a theory.
Similarity Matching: This may also be referred to as Association; Seeking similarities is perhaps most often used to detect anomalies or outliers. Remember, this is where the system learns without the users inputting which independent variables are likely to affect the dependent variable. A typical use case might be to identify which customers are likely to cancel an ongoing service or order a particular item or set of items. Or which suppliers are likely to miss their due date to your facility. This can also indicate auto-approval for all of the forecasts or replenishment plans that are similar to a usual pattern, and alert users when they are likely to want to review a plan.
Clustering: This method leads to groupings of objects that are more similar to each other than those in other clusters. A typical use case is to identify natural groups for suppliers or for customers. Clustering might also improve the accuracy of a supervised ML method, such as classification. In this case, it might apply to products, creating a different breakdown than the currently identified A, B, C items, as the system can identify other natural groupings.
Oops! We could not locate your form.